Dr. Massimo Domenico Sammito, Senior Computational Scientist, PhoreMost
Recent developments in Artificial Intelligence (AI) have led to a revolution in many scientific branches, including drug discovery and structural biology. The Cambridge biotech and pharma ecosystem has embraced such advances by introducing and developing ad-hoc AI strategies to accelerate and lead the market from discovery and through pre-clinical to phase III clinical stages. The University of Cambridge, in its efforts to help bridge the gap between industry and academia, recently hosted the first Cambridge Conference of AI in Drug Discovery. I was lucky enough to attend the virtual event as a representative from PhoreMost. We are exploring the combination of AI with our phenotypic screening platform to more efficiently identify a broad range of novel targets and to discover lead compounds and translate them into high-quality first-in-class assets.
The conference provided a comprehensive overview of both scientific research transforming drug discovery as well as insights from some of the most innovate entrepreneurs in the field. Instrumental to this and cited by Forbes as “the most important achievement in AI – ever”, is AlphaFold2, DeepMind’s AI system for predicting the 3D structure of proteins, debuted at CASP14, 14th Critical Assessment of Protein Structure Prediction. The extraordinary results achieved by Deepmind’s AlphaFold2 algorithm were and still are really incredible. For the first time, in silico predictions of monomeric globular protein structures were comparable to high-resolution experimental results. The latest version of AlphaFold has already influenced the drug development industry by accelerating structural studies that aim to identify drug interactions with protein targets. The system has even shown promise that protein structure prediction could be beneficial in future pandemic preparedness – AlphaFold was able to predict several protein structures of the SARS-CoV-2 virus.
Particularly impressive was the presentation given by Prof. Michael Bronstein, DeepMind Professor of AI at the University of Oxford, who introduced the latest developments in the field of Graph Neural Networks – architecture that enables the description of complex multidimensional objects, such as molecules or biological pathways, as Graphs composed of connecting Vertices and Edges (Figure 1).
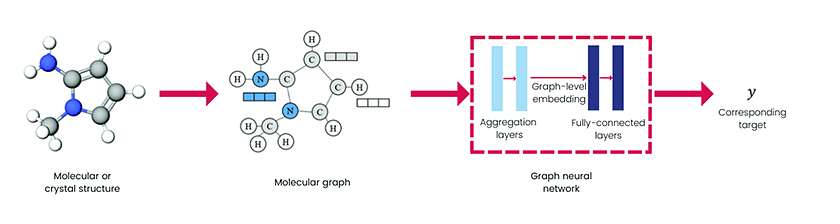
Figure 1: Graph Neural Networks (redrawn from G.S. Na, H. Chang, H.W. Kim; PCCP, 2020)
Prof. Bronstein demonstrated how the graph could readapt and “rewire”, dynamically modifying the connections in such a way to optimise the “flux” of information passed across the network. With this powerful algorithm, it is possible to influence very distant nodes in the network. This feature is important in the modelling of biomolecular structures where local modifications in one region lead to large movements in another region of the structure.
Another key discussion point was the importance of improving technical skills to support the implementation of cutting-edge AI algorithms and applying them in real scientific applications. This is paired with not losing track of the overall picture and understanding the dynamics of the market related to AI within drug discovery. Dr Andreas Bender, CSO of Terra Lumina and Co-Founder of Healx, explained how an increase of investment in a sector might produce a “bubble”. We are currently on the “AI-hype”, but there is increasing pressure for the technology to deliver qualified and non-obvious outcomes to justify the amount of money invested. Results cannot be obtained quickly due to the nature of the problem we are trying to address with AI. This technique is based on a large amount of solid and validated data that is not necessarily available for all drug discovery applications. Moreover, the input context that we can describe and the process for an AI approach is too limited, simplistic, or approximated and does not completely reflect the real implications and interconnections. AI in this area is still challenging and something that requires strong expertise, as well as dedicated internal development. Nevertheless, outstanding results are being announced, for example, the discovery of powerful antibiotics against ‘untreatable’ bacteria strains. Although at times slow, exciting discoveries help maintain optimism in the community towards a synergistic growth of AI technology and drug discovery programmes.
At PhoreMost, we are dedicated to drugging so-called undruggable targets using our next-generation technology, SITESEEKER®, which systematically identifies cryptic drug sites throughout the human proteome and ties them to useful therapeutic functions. AI, combined with phenotypic screening, offers an exciting possibility of further expanding the druggable space and is already having a substantial impact in virtual screening and drug modelling. Already, through a drug discovery collaboration with XtalPi, an AI leader with operations in China and the US, we are harnessing the technology to identify and investigate targets that epigenetically regulate tumour progression and have been previously classified as undruggable. This approach promises to quickly identify and translate lead compounds with desirable drug properties.
AI is a powerful tool to be used within drug discovery. However, the associated scientific criticism surrounding the hypothetical results should be taken into account, leading to subsequent testing and experimental confirmation. PhoreMost’s approach is to use sophisticated algorithms and Deep Neural Network architectures to better interpret our experimental data, to enlighten on emergent properties that can lead to new and exciting discoveries. The internal Bioinformatics/Data Science team aims to reduce the gap between experimentalists and computational scientists developing a data cycle in which experiments, related statistics and their visualisations lead to the prediction and inferring of new insights that are then fed again into new experimental projects. In this way, harnessing AI and machine learning can support our investigations into expanding druggable space.
At PhoreMost, we are expanding our computational research team – if you are interested in the work we’re doing and this innovative application, please visit phoremost.bamboohr.com/jobs for more information, or get in touch!
Contact: info@phoremost.com